Algorithms could create an even playing field—if we insist on it
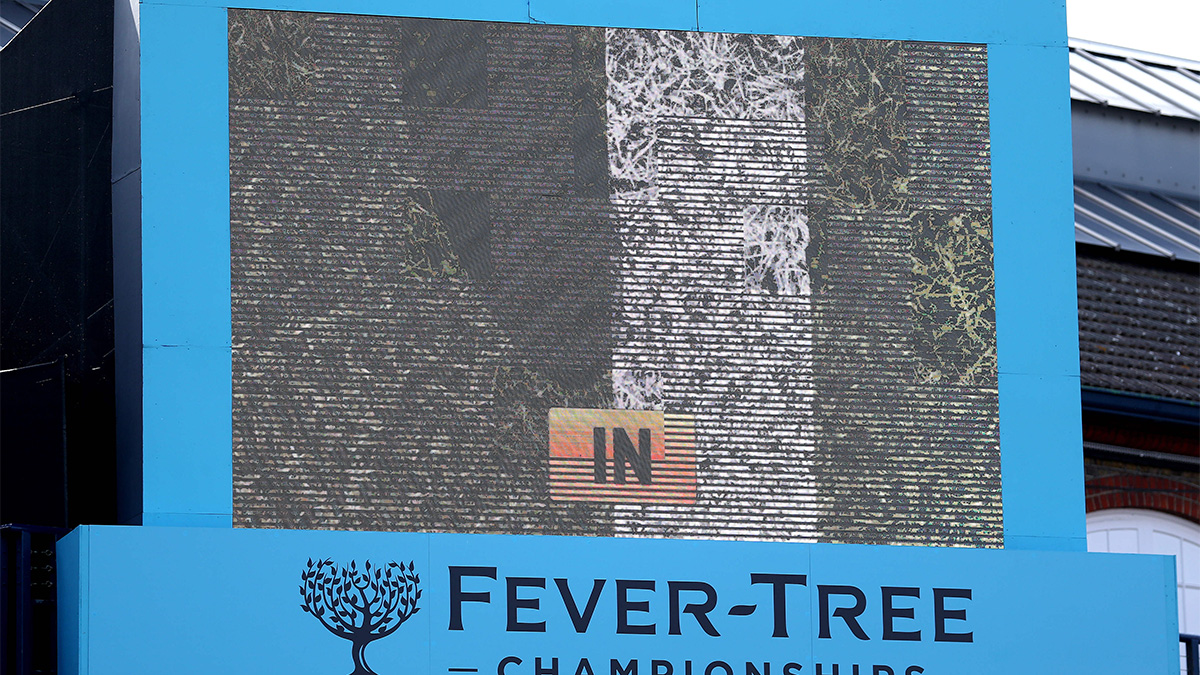
Each month in 2018, Future Tense Fiction—a series of short stories from Future Tense and ASU’s Center for Science and the Imagination about how technology and science will change our lives—will publish a story on a theme. The theme for July–September 2018: Sport.
An expert on algorithmic bias responds to Deji Bryce Olukotun’s “When We Were Patched.” This column originally appeared in Slate and is used with permission.
Big decisions about our lives are increasingly made jointly by humans and computer systems. Do we get a loan? Are we invited for an interview? Who should we date? Which news stories should we read? Who won the tennis match? This is our reality today. In “When We were Patched,” Deji Olukotun explores what the boundaries of these human and machine partnerships will be. Could we get the best of both, or will we end up with the worst of both?
Humans, we like to believe, have common sense and intuition, while computers are logical and cold. However, in “When We Were Patched,” Olukotun challenges these assumptions. It is the computer’s feelings that are hurt by the impatience and disregard of Malik, the human partner in officiating a championship FogoTennis match. The computer officiant, Theodophilus Hawkeye the Sixteenth, is the one who is eager to please, sensitive to the emotional cues of the human, jilted, and resentful after their partner causes them to be fired. We even hear the computer declare that officiating is woven into its very soul.
In Olukotun’s story, when humans and computers partner to make decisions, it is the A.I. who is blamed for perceived errors. Systems could also be designed to absorb blame, relieving human decision makers of the karmic cost of making difficult choices, like whom to fire or whom to send to prison, leaving humans to declare, “I just do what the computer tells me.” Alternatively, in her 2016 paper “Moral Crumple Zone: Cautionary Tales in Human Computer Interaction,” Madeleine Clare Elish, my colleague at the Data and Society Research Institute, describes how human pilots were blamed after they failed to recover from a stall when the autopilot system shut itself off, causing the fatal crash of Air France Flight 447. Elish argues that an increase in automation can make pilots’ skills atrophy, while simultaneously asking them to absorb legal and moral liability when automated systems fail. What freedom will even doctors or judges have to “overrule” the computer without risking, for example, malpractice suits or a record that looks “soft on crime?” Whose judgment can be challenged and in what ways?
In tennis’s “gentlemanly” culture, the idea of challenging an umpire chair used to be deeply offensive. But in 2001, the Hawk-Eye instant-review system was introduced into professional tennis, and challenges to rulings on the court became commonplace. Theodophilus accuses Basto of using challenges in an “unsporting” manner, but Hawk-Eye challenges today are widely accepted because strategies around challenges make the game more entertaining for audiences. They provide a way to interrupt a dominating player’s momentum, heighten the stakes of the game at crucial turning points, and build mounting suspense in the audience during a slow-motion replay of the shot. They also frequently show the human umpire wrong—one report found that the system upheld the player’s challenge 45 percent of the time.
On the other hand, there is a pervasive myth that decisions made by computers are fundamentally logical, unbiased, almost infallible. That’s not true. Automated decision making is often just plain wrong—and wrong along familiar historical lines of inequality. Researchers Joy Buolamwini and Timnit Gebru found that some commercial-grade facial recognition software had a 34.7 percent error rate for dark-skinned women, but only a 0.8 percent error rate for light-skinned men. Further, computers are built by humans, and their decisions reflect structural inequalities and human bias, just in different, subtle ways. For example, machine learning algorithms are “trained” on data sets that reflect how people have made decisions in the past. They ask what patterns have predicted past success and so they learn to prefer the types of people who have been successful in the past. In other words, they become the perfect gatekeepers of the establishment—that may be how Theodophilus Hawkeye the Sixteenth, a “purebred” machine, comes to favor the masculine Isle of Man player with “royal blood” when Malik actively roots for Basto, the underdog from Macau, likely a young woman of color, competing aggressively against a veteran player.
In Olukotun’s story, Malik calls for a “full audit of Theodophilus’ source code.” But the answer there may not be straightforward—and if there is bias in an A.I. referee system, it could be intentional or not. For instance, a programmer could write a program that reflected specific bias (for instance, a line of code that says “if the applicant is a woman, deduct 10 points from her score”). It could also have happened in other ways. Automated systems could learn ugly behavior by observing people, as in the case of Microsoft’s Tay bot—which began parroting racist, misogynistic speech in less than 24 hours of being trained online by internet users—or included by a machine-learning algorithm that uses a set of data to teach itself (such as figuring out how to spot good CEOs to hire by looking at a pool of people who are successful CEOs today). Every training set will include historical value judgments of what has been considered “good” and “successful.” What gets baked in with those assumptions if our historical patterns reflect structural inequality? Those kinds of biases would not easily be audited in the source code alone.
Anyway, an audit would likely require permission from FogoTennis and the companies that built Theodophilus, and that may be difficult to obtain. Legal scholar Rebecca Wexler examines how trade secret protection is regularly used to shield bad algorithmic decision making from review even in crucial areas of public concern like criminal justice where the intellectual property rights of software developers are routinely prioritized over a defendants’ rights to understand and examine the evidence against them. In 2017, public policy committees with the influential Association for Computing Machinery issued a statement outlining ethical principles for algorithmic accountability and transparency. They called on designers to build automated systems that provide explanation, verification, testing, and the ability to question decisions made. Without a commitment to accountability, transparency, and iterative improvement, we are doomed to continue to err in the same ways humans already have. It is difficult to imagine an automated system challenging historical social norms unless it was directed to do so. Automated systems can even amplify those errors, for example in the case of predictive policing algorithms that not surprisingly find more crime in neighborhoods to which more police are dispatched.
In “When We Were Patched,” Malik asks whether speed or power make for a better athlete. The better question might be, who is the game designed to advantage? Olukotun’s story challenges us to consider what is possible if a society decided it wanted to change the conditions of the game, developing new technologies that would create the conditions for coed tennis in which players muscular and powerful, slim and speedy could test their powers against one another. Theodophilus Hawkeye the Sixteenth is unable to answer Malik’s question, but neither could we. The answer can’t be derived from the past alone: It depends on what we collectively decide about the future, about what justice looks like, about leveling the playing field in sports and in life. As in Olukotun’s story, humans and computers will be working together to pick winners and losers. We need to collectively decide on and enforce the rules they will follow. We need the ability to understand, challenge and audit the decisions. A level playing field won’t be the future unless we insist on it.
Jeanna Matthews is a computer science professor at Clarkson University and fellow at the Data and Society Research Institute.
Data and Society research analyst Kinjal Dave contributed research and ideas to this article.
Future Tense is a partnership of Slate, New America, and Arizona State University that examines emerging technologies, public policy, and society.